Generative AI has taken over the world. Beyond the hype and the possibly over-inflated expectations that it can yield in the short term, generative artificial intelligence (gen AI) represents the beginning of a paradigm shift that changes not only how machines are programmed, but also how they are built. What was once a co-processing unit that assisted the CPU with graphics tasks has evolved into a massively parallel compute powerhorse that is now at the core of the generative AI revolution.
A previous article describes how to fine-tune Llama 3.1 models on OpenShift AI using Ray and NVIDIA GPUs. This article covers the latest generation of AMD GPUs, specifically AMD Instinct MI300X accelerators, and reviews the progress that has happened recently in the open source ecosystem that makes it now possible to run state-of-the-art AI/ML workloads using ROCm, AMD’s open source software stack for GPU programming, on Red Hat OpenShift AI.
Note
Catch up on the series so far:
- How to fine-tune Llama 3.1 with Ray on OpenShift AI
- How AMD GPUs accelerate model training and tuning with OpenShift AI
- How to use AMD GPUs for model serving in OpenShift AI
The road to AMD GPU support in OpenShift AI
An entire stack is needed for fine-tuning large language models, such as Llama 3.1 models on OpenShift with AMD accelerators support, as illustrated in Figure 1.

At the operating system level, the AMD GPU driver and ROCm 6.x libraries have already supported Red Hat Enterprise Linux (RHEL) 9.x for some time.
At the container orchestration layer, a lot of work has happened over the last few months in the community AMD GPU operator. Future work on the AMD GPU operator will be addressed and officially supported by AMD.
At the application layer, while PyTorch support for AMD accelerators was introduced in version 2.0 last year, it’s only very recently that a critical mass has been reached in the ecosystem. In particular:
- Ray, which got proper ROCm integration—one that calls the ROCm SMI library directly—released in version 2.30.0 on June 21, 2024.
- Flash Attention, which recently received ROCm support, released in version 2.3.6 on July 25, 2024.
- DeepSpeed, which had advertised ROCm integration for quite some time but which needed some fixes to skip the compilation of incompatible optimizers (not supported by ROCm), released in version 0.15.1 on September 5, 2024.
As part of the developer preview for AMD GPU support in the OpenShift AI tuning stack, Red Hat published a container image at quay.io/rhoai/ray:2.35.0-py39-rocm61-torch24-fa26 that packages pre-compiled Flash Attention and DeepSpeed compatible with ROCm 6.1.
Portability over accelerators
In the daily routine of a data scientist, what matters is experimenting with the libraries that you like to get your job done as quickly as possible. At equivalent performance levels, it shouldn’t matter what kind of accelerators are behind the scene to run your AI/ML workloads.
At the level of the tuning stack in OpenShift AI, the changes required to port the fine-tuning Llama 3.1 with Ray on OpenShift AI example from one accelerator to the other are minimal.
# Configure the Ray cluster
cluster = Cluster(ClusterConfiguration(
name='ray',
namespace='ray-finetune-llm-deepspeed',
num_workers=7,
worker_cpu_requests=16,
worker_cpu_limits=16,
head_cpu_requests=16,
head_cpu_limits=16,
worker_memory_requests=128,
worker_memory_limits=256,
head_memory_requests=128,
head_memory_limits=128,
# Use the following parameters with NVIDIA GPUs
# image="quay.io/rhoai/ray:2.35.0-py39-cu121-torch24-fa26",
# head_extended_resource_requests={'nvidia.com/gpu':1},
# worker_extended_resource_requests={'nvidia.com/gpu':1},
# Or replace them with these parameters for AMD GPUs
image="quay.io/rhoai/ray:2.35.0-py39-rocm61-torch24-fa26",
head_extended_resource_requests={'amd.com/gpu':1},
worker_extended_resource_requests={'amd.com/gpu':1},
))
Et voilà! Assuming you are connected to an OpenShift cluster with AMD Instinct accelerators, you can follow the steps in the previous post and only change these three lines to configure your Ray cluster; the configuration for fine-tuning Llama 3.1 models stays exactly the same:
image="quay.io/rhoai/ray:2.35.0-py39-rocm61-torch24-fa26",
head_extended_resource_requests={'amd.com/gpu':1},
worker_extended_resource_requests={'amd.com/gpu':1},
Figure 2 shows the results produced during validation tests for a distributed job that fine-tuned Llama 3.1 8B model on the GSM8K dataset, with 4x AMD MI300X accelerators, LoRA, and a 4K context length. It shows typical convergence, with the training and evaluation losses quickly decreasing and then asymptotically converging to their local minima, while the average forward and backward pass durations are stable.

The runtime metrics from the AMD Instinct accelerators aren’t yet integrated into the OpenShift monitoring stack. To view the real-time usage during fine-tuning, you can run the ROCm CLI inside one of the worker pods by opening a terminal from the OpenShift web console, as shown in Figure 3.
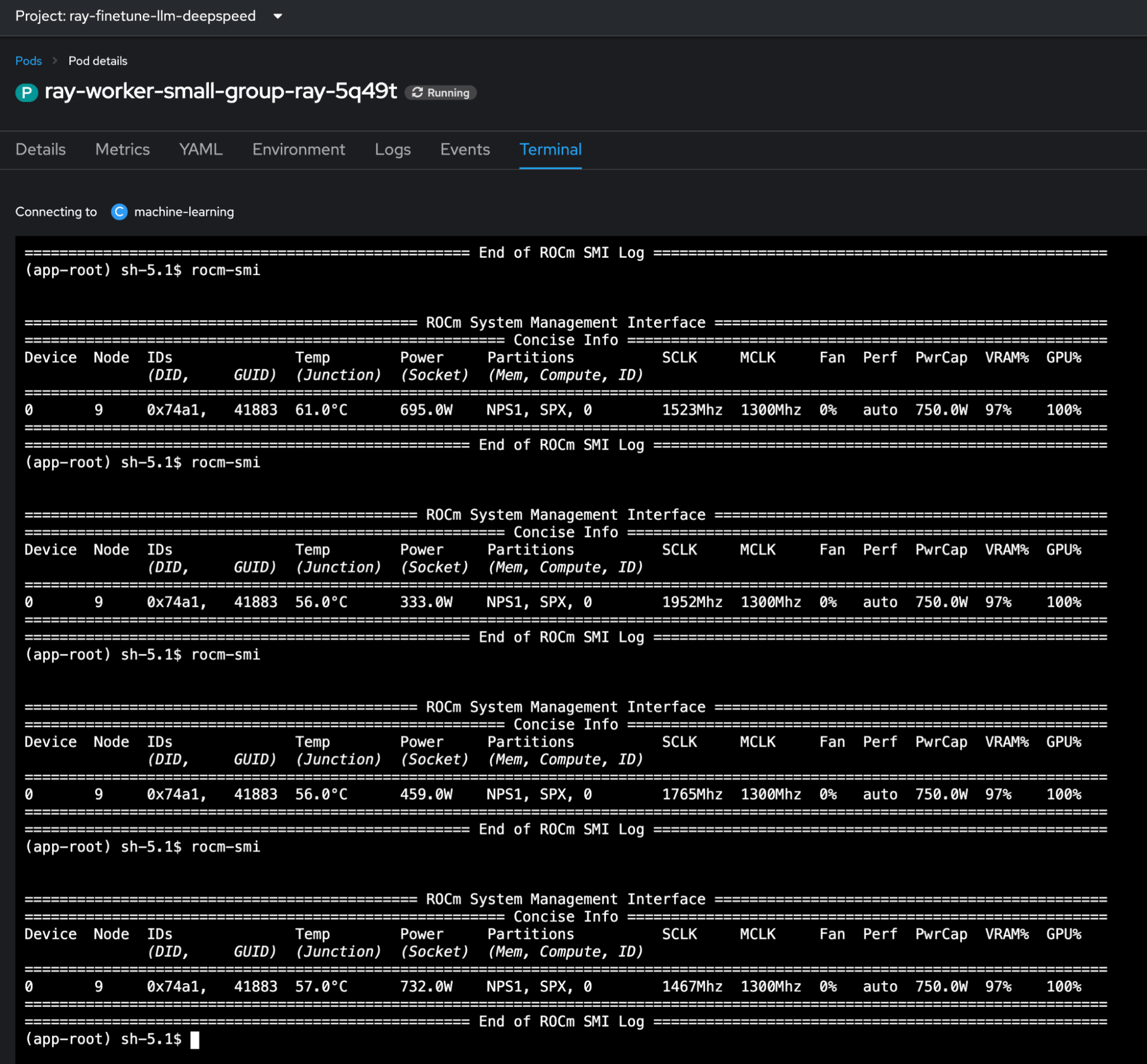
Conclusion
Earlier this year, AMD and Red Hat announced their collaboration to expand customer choice for building and running AI workloads. The work delivered by Red Hat, AMD and the larger community makes it now possible to run state-of-the-art AI workloads on the latest generation of AMD accelerators.
AMD Instinct MI300X, with its 192GB of high-bandwidth memory, is a game-changer for running workloads where memory size and bandwidth is often the bottleneck, such as when training and serving LLMs.
Experiments with fine-tuning Llama 3.1 with Ray on OpenShift AI demonstrate that you can significantly reduce fine-tuning duration by increasing batch sizes, or reach longer context lengths thanks to this larger memory size.
In the next article in this series, Vaibhav Jain will guide you through how to integrate and utilize AMD GPUs in OpenShift AI for model serving: How to use AMD GPUs for model serving in OpenShift AI
Also, be sure to visit the Red Hat OpenShift AI product page to learn more about how you can get started.
Last updated: October 18, 2024